Overview
The goal of this database is to share in vivo medical images of patients wtith brain tumors to facilitate the development and validation of new image processing algorithms.
Pre- and post-operative MR, and intra-operative ultrasound images have been acquired from 14 brain tumor patients at the Montreal Neurological Institute in 2010. All patients signed a specific consent form for the distrbution of their anonymized images online (NEU-09–010). Each patient had a pre-operative and a post-operative T1-weighted MR with gadolinium and multiple B-mode images pre- and post-resection. Corresponding features were manually selected in some image pairs for validation. All images are in MINC format, the file format used at our institute for image processing.
The images were acquired with our prototype neuronavigation system IBIS NeuroNav by two neurosurgeons: Dr Rolando Del Maestro and Dr Kevin Petrecca wth the help and training of PhD student Laurence Mercier. Neurosurgeon Dr Claire Haegelen and neuroradiologist Dr David Araujo helped selecting manual tags for validation. The principal investgator of this project is Dr Louis Collins.
While this page is under construction, the data is available below.
References
If you use images from our database please cite the following paper:
L. Mercier, R.F. Del Maestro, K. Petrecca, D. Araujo, C. Haegelen, D.L. Collins. On-line database of clinical MR and ultrasound images of brain tumors. Med Phys. 2012 Jun;39(6):3253–61. (link in PubMed)
Data
Some of the images provided have already been used for earlier publications. For that reason, the data are divided in 3 groups with their own characteristics and features.
Group 1: Pre- and post-resection ultrasound images
This group contains 2D and 3D ultrasound images before and after tumor resection. For each of the 14 patients, more than one sweep was acquired pre- and post-resection. One of the pre- and one of the post-resection sweeps were chosen to form a pair for which 10 homologous landmarks were chosen by neuroradiologist Dr David Araujo. These landmarks can be used to compute the distance between the 2 images for the validation of registration algorithms.
Download Group1 data (4.6G) Readme file
Group 2: Pre-operative MRI and pre-resection ultrasound images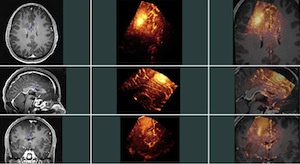
This group contains 14 pairs of pre-operative MR and pre-resection ultrasound images (2D and 3D). For each MRI-3DUS pair, homologous landmarks were chosen by 2 experts (Dr Louis Collins and Laurence Mercier). The 6 first patients were also tagged by a third expert, neurosurgeon Dr Claire Haegelen. These landmarks can be used to compute the distance between the 2 images for the validation of registration algorithms. In this group, both the MR and ultrasound images were transformed into the MNI Talairach-like brain-based coordinate space (orientation and position only, no scaling), as the manual taggers found it easier to visualize the MRI in that frame of reference.
Download Group2 data (2.1G) Readme file
Group 3: Pre- and post-resection MR images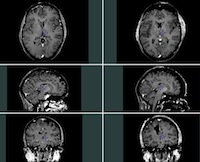
This section includes MR images taken at two different time points: before and after surgery. The images in this group have not previously been used for a publication and do not therefore have any tags available for registration validation. The MRI are provided in their original scanner frame of reference.
Download Group3 data (565M) Readme file
Group 4: Pre-operative MR and post-resection US images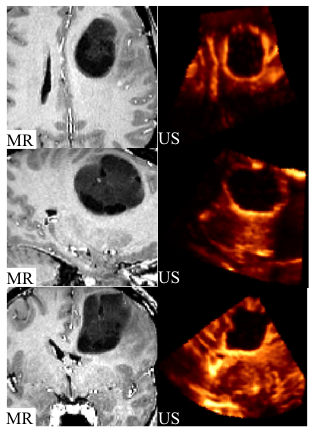
This group includes pre-operative MR and intra-operative post-resection US images. Data in both MINC and mat (MATLAB) format is provided for all patients. The pre-operative MR data contains the tumour, which is replaced by the resection cavity in the intraoperative US images. Since the tumour in the pre-operative MR does not exist in the post-resection US images, registration of these images is very challenging. Corresponding homologous landmarks are selected in US and MR images for validation of image registration algorithms (15 points in average per patient). Landmarks are selected twice in 6 patients to measure the reproducibility of landmark selection (see the paper below). Please cite the following paper if you use this data:
Rivaz, H., Chen, S, Collins, DL., Automatic Deformable MR-Ultrasound Registration for Image-Guided Neurosurgery, IEEE Trans. Medical Imaging, 2015, 34(2): 366–380 (link in PubMed)
Download Group4 data (591M) Readme file
General instructions: Once you have saved the data on your computer here’s what you need to do do detar and unzip it in Linux: gunzip group?.tar.gz tar -xvvf group?.tar Then please have a look at the accompanying readme files for more information.
A few tips:
- Files with the extension .mnc are the image files and those with the extension .tag are text files containing the manually selected homologous landmarks.
- The easiest way to visualize one or two MINC images is to use register. To view a pair of images along with their tags use the following command:
register image1.mnc image2.mnc landmarks.tag
- Files with the extension .xfm are text files containing transformations. To apply a transformation on a .mnc file, use the tool mincresample. To apply a transformation on a .tag file, use the tool transform_tags
- To extract the parameters (translations, rotations, etc) from a linear transformation stored in a .xfm file, use: xfm2param.
- To reconstruct a sweep of 2D images into a 3D volume use volregrid. For example, the command that was used to reconstruct the 3D ultrasounds in this study is the following:
volregrid `volextents -step 0.3 *.mnc -regrid_floor 0.0001 -linear -regrid_radius 0.3 0.3 0.3 -xdircos 1 0 0 -ydircos 0 1 0 -zdircos 0 0 1 *.mnc 3dus.mnc
- volextents is not part of the standard MINC distribution, but you can download it here.
- For each 3D ultrasound provided on this web site, the corresponding 2D slices are also available, which means that you can reconstruct your own 3D ultrasound with, for example, a different resolution.