At the NIST we work on a number of different medical image visualization problems, to make important information visible and salient to the viewer. Projects include:
Normal Aging
Over the past several years we have collaborated with several individuals to study normal aging. Using Alzheimer’s disease, amnesic mild cognitive impairment and healthy individuals we have demonstrated that interhemispheric coupling may be regarded as a flexible mechanism that can improve the brain’s ability to meet processing demands for high cognitive demand in normal aging and for low cognitive demand in AD [1].
In a recent study the links between cognitive ability and cortical tissue volume in old age were investigated. Evidence from this research warns against an exclusive reliance on the causal link between cognitive function and the cortical tissue in old age based on assumptions of the aging process. Preservation of cortical tissue thickness in old age is not a foundation for successful cognitive aging, but rather reflects a lifelong association [2].
Reference
[1] J. Ansado, D.L. Collins, S. Joubert, V.S. Fonov, O. Monchi, S.M. Brambati, F. Tomaiuolo, M. Petrides, S. Faure, Y. Joanette, Yves. “Interhemispheric coupling improves the brain’s ability to perform low cognitive demand tasks in Alzheimer’s disease and high cognitive demand tasks in normal aging”, Neuropsychology, Vol 27(4), Jul 2013, 464–480.
[2] Karama S, Bastin ME, Murray C, Royle NA, Penke L, Muñoz Maniega S, Gow AJ, Corley J, Valdés Hernández Mdel C, Lewis JD, Rousseau MÉ, Lepage C, Fonov V, Collins DL, Booth T, Rioux P, Sherif T, Adalat R, Starr JM, Evans AC, Wardlaw JM, Deary IJ. Childhood cognitive ability accounts for associations between cognitive ability and brain cortical thickness in old age. Mol Psychiatry. 2014 May;19(5):555–9.
Augmented Reality in Neurovascular Surgery
In neurovascular surgery, and in particular surgery for arteriovenous malformations (AVMs), the surgeon must map pre-operative images of the patient to the patient on operating room (OR) table in order to understand the topology and locations of vessels below the visible surface. This type of spatial mapping is not trivial, is time consuming, and may be prone to error. Using augmented reality (AR) we can register the microscope/camera image to pre-operative patient data in order to aid the surgeon in understanding the topology, the location and type of vessel lying below the surface of the patient. This may reduce surgical time and increasing surgical precision. In this project as well as studying a mixed reality environment for neuromuscular surgery, we will examine and evaluate which visualization techniques provide the best spatial and depth understanding of the vessels beyond the visible surface.
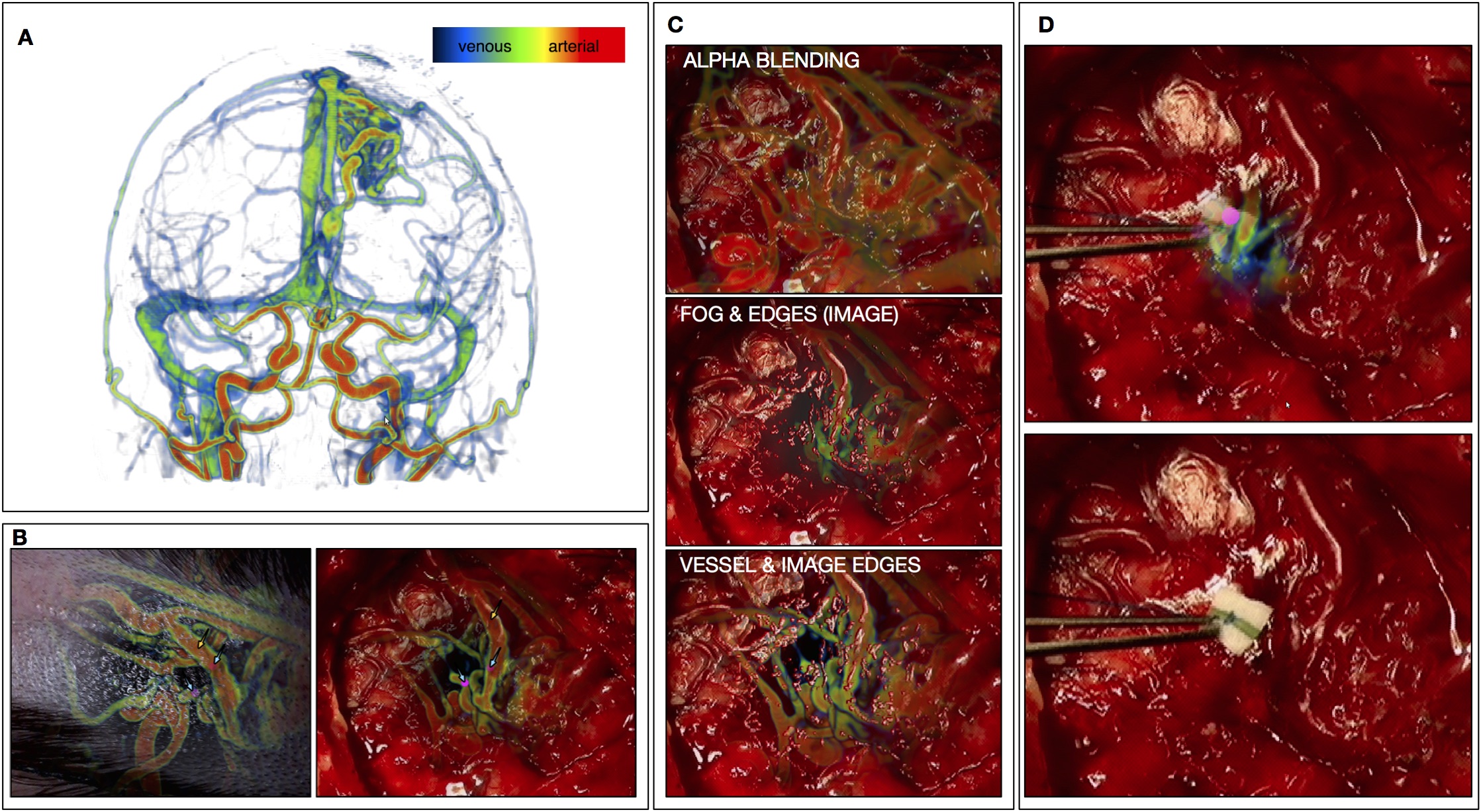
A: Colour coding of a vascular DS-CTA volume based on blood flow. B: Vessels overlaid on the patient skin prior to draping (left). The AR view is used at this step to help tailor the extent of the craniotomy. On the right we see vessels overlaid on the cortex prior to resection, here the AR view is used to determine the optimal resection corridor. The blue arrows point to the pink markers that indicate the location of deep feeding arteries. The orange arrow indicates the major arterialized vein, shown as red and not blue. C: Different visualization techniques for combining the live camera image (prior to resection) with the virtual vessels (green, red, blue) are shown.The use of simple alpha-blending between the real and virtual worlds does not provide spatial information (top). More sophisticated techniques such as modulating transparency in the area of interest and using edges (from the virtual vessels and/or camera image) and using fog are applied. D: Based on the virtual information the surgeon placed a micropad on the brain surface above a virtual marker representing a deep feeding artery to help with the resection approach and vessel localization.
Publications
M. Kersten-Oertel, M., Gerard, I., Drouin, S., Mok, K., Sirhan, D., Sinclair, D. S. and Collins, D. L. Augmented reality in neurovascular surgery: feasibility and first uses in the operating room. IJCARS (2015): 1–14.
Kersten-Oertel, M., Gerard, I. J., Drouin, S., Mok, K., Sirhan, D., Sinclair, D. S., & Collins, D. L. (2015). Augmented Reality for Specific Neurovascular Surgical Tasks. In Augmented Environments for Computer-Assisted Interventions (pp. 92–103). Springer International Publishing.
M. Kersten-Oertel, I. Gerard, S. Drouin, K. Mok, D. Sirhan, D. Sinclair, D. L. Collins. “Augmented Reality in Neurovascular Surgery: First Experiences.” Augmented Environments for Computer-Assisted Interventions. Lecture Notes in Computer Science Volume 8678, 2014, pp 80–89, 2014.
Augmented Reality
In image-guided surgery the surgeon must map pre-operative patient images from the navigation system to the patient on operating room (OR) table in order to understand the topology and locations of the anatomy of interest below the visible surface. This type of spatial mapping is not trivial, is time consuming, and may be prone to error. Using augmented reality (AR) we can register the microscope/camera image to pre-operative patient data in order to aid the surgeon in understanding the topology, the location and type of vessel lying below the surface of the patient. This may reduce surgical time and increasing surgical precision.
Our current projects in this area include:
Alzheimer’s
Alzheimer’s disease (AD) is a progressive neurodegenerative disease, which is the most common cause of dementia. Its prevalence for people aged 65-70 y is 1%, while it is 7% for the 75-84 y group, and 26% among those aged 90 and older. In Canada, persons over age 65 will make up to 15% of the population by 2016, but this amount is estimated to reach 23% by 2041. It is assumed that the prevalence of AD will quadruple by 2050 resulting in great financial burden.
It is believed that the pathophysiological process of AD begins well before the diagnosis of the dementia. Like many other neurodegenerative diseases, early treatment, before occurrence of too much irreversible degeneration of brain tissue, can be more effective. However, early diagnosis of Alzheimer’s disease is currently almost impossible. The well-cited biomarker model that the structural MRI begins showing abnormality at the preclinical stage and rises significantly in MCI stage, which makes it an interesting candidate for prognosis of dementia onset. Our group with sophisticated image analysis techniques followed by statistical analysis tries to make the diagnosis and prediction possible at the level of an individual person.
Projects include:
White Matter Hyperintensities
Neurodegenerative diseases such as Alzheimer’s disease (AD) commonly coexist with cerebrovascular disease in the elderly population. Cerebral small vessel disease (SVD) is the most common vascular cause of dementia and a major contributor to mixed dementia. SVD frequently coexists with AD and can increase the cognitive and physical deficits caused by neurodegeneration. White matter hyperintensities (WMHs) are considered to be one of the major signs of SVD on MRI and are associated with neurological and cognitive symptoms and physical difficulties. We have developed automated tools for segmentation of WMHs in Alzheimer’s patients using multiple contrasts of MR images. We use these segmentations to study the effect of WMHs in AD.
WMH Segmentation Pipeline:
https://www.dropbox.com/sh/zbbqjjo1ilzuun2/AABWN17N2fyzi8p3aSfiA0fEa?dl=0
Docker Container:
docker pull nistmni/wmhchallenge_2017
Execution method:
CONTAINERID=$(docker run -dit --name wmh_test -v
$(pwd)/Docker_Files/${input}/orig:/input/orig:ro -v
$(pwd)/Docker_Files/${input}/pre:/input/pre:ro -i -v /output
nistmni/wmhchallenge_2017 )
docker exec $CONTAINERID "/home/nistmni/run.sh"
docker cp $CONTAINERID:/output result
docker stop $CONTAINERID
docker rm -v $CONTAINERID
Journal Publications:
- Dadar, M., Maranzano, J., Ducharme, S., Collins, D. L., & Alzheimer’s Disease Neuroimaging Initiative. (2019). “White Matter in Different Regions Evolve Differently During Progression to Dementia”. Neurobiology of Aging.
- Dadar M., Zeighami Y., Yau Y., Fereshtehnejad S.M., Maranzano J., Postuma R.B., Dagher A., Collins D.L. (2018), “White Matter Hyperintensities Are Linked to Cognitive Decline in de Novo Parkinson’s Disease Patients”. NeuroImage: Clinical, 20, 892-900.
- Dadar, M., Fonov, V. S., Collins, D. L., & Alzheimer’s Disease Neuroimaging Initiative. (2018). A comparison of publicly available linear MRI stereotaxic registration techniques. NeuroImage, 174, 191-200.
- Dadar, M., Maranzano, J., Ducharme, S., Carmichael, O. T., Decarli, C., Collins, D. L., & Alzheimer’s Disease Neuroimaging Initiative. (2018). Validation of T 1w‐based segmentations of white matter hyperintensity volumes in large‐scale datasets of aging. Human brain mapping, 39(3), 1093-1107.
- Dadar, M., Maranzano, J.,…, Collins, D.L. & Alzheimer’s Disease Neuroimaging Initiative. (2017). Performance comparison of 10 different classification techniques in segmenting white matter hyperintensities in aging. NeuroImage, 157, 233-249.
- Dadar, M., Pascoal, T. A., Manitsirikul, S., Misquitta, K., … & Collins, D. L. (2017). Validation of a regression technique for segmentation of white matter hyperintensities in Alzheimer’s disease. IEEE transactions on medical imaging, 99, 1-1.